CMU team succeeds in 7th Crystal Structure Prediction Blind Test
Kaitlyn Landram
May 31, 2023
A common practice in industrial sectors that produce molecular materials is crystallizing molecules into solid forms to achieve uniform and reproducible properties. The structure of these molecular crystals directly impacts their properties and dictates the end use of the material. For example, the crystal structures of pharmaceuticals can impact their solubility, bioavailability, and efficacy, making molecular crystallization a key aspect of the drug development process. Because molecular crystals are bound by weak interactions the complication of polymorphism arises, where the same molecule may crystallize into more than one structure. The appearance of unexpected polymorphs in the pharmaceutical manufacturing process can create disastrous consequences, as it can render a drug ineffective and even unsafe. One example of this was seen with Ritonavir, an antiviral drug to treat HIV, which was ultimately pulled from the market following the appearance of a less soluble polymorph that resulted in it being medically useless. It is estimated that this incident cost Abbott Laboratories around $250 million. Crystal structure analysis is an integral part of a drug’s formulation and is critical to ensuring a target compound will produce the desired structure. Predicting possible polymorphs enables industries to carry out risk assessment and proper efficacy testing before production.
Identifying the expected crystal structures from the knowledge of the molecular structure defines the crystal structure prediction challenge. This is a highly nontrivial task because, first, the energy differences between polymorphs can be miniscule, requiring very high accuracy, and second, the number of possible structures is immense, requiring high efficiency. In 1999, the Cambridge Crystallographic Data Center developed the Crystal Structure Prediction (CSP) Blind Test as a way to bring together leading scientists from industry and academia to assess the progress of CSP methods. Participants in the test are given a 2D “stick diagram” of a molecule and have to use computer simulations to predict its crystal structure. The 7th CSP Blind Test comprised two phases. The first phase tested participants’ ability to generate the correct crystal structure and the second phase tested participants’ ability to correctly rank structures from most to least stable at room temperature.
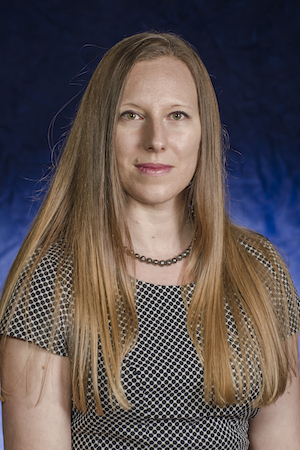
Noa Marom
Noa Marom, Associate Professor of Materials Science and Engineering, and Olexandr Isayev, Assistant Professor of Chemistry, led the CMU team that included Marom’s Ph.D. students: NSF Fellow Imanuel Bier (MSE), MolSSI Software Fellow Rithwik Tom (Physics), DOE SCGSR Fellow Dana O’Connor (MSE), and MSE MS students: Yi Yang, Kehan Tang, and Wenda Deng, as well as Isayev’s lab members: Dr. Roman Zubatiuk (Chemistry), Dr. Dylan Anstine (Chemistry), and Chemistry Ph.D. students: Kamal Nayal and Shuhao Zhang.
The team combined quantum mechanical simulations, optimization algorithms, and machine learning to perform CSP. Marom’s structure generation codes were used in conjunction with Isayev’s neural network interatomic potentials. During the test, they developed novel computational methodology for structure generation and for training system-specific machine learned interatomic potentials. These potentials are crucial for the acceleration of geometry optimization and stability evaluation for millions of generated structures. Their codes will be widely available to researchers across the globe advancing crystal structure prediction.
In the first phase, the CMU team successfully generated the known polymorphs of two out of three attempted targets: an organic electronic material (Target XVII) and an agrochemical compound (Target XXXI). Target XVII was particularly challenging because the molecule has flexible side chains that can assume a very large number of conformations. The CMU team generated and evaluated 5 million structures of this target and was one of only three teams to predict the correct structure with an average deviation of less than 0.5 Å from experiment. For Target XXXI, the CMU team generated and evaluated 1.7 million structures. The team correctly predicted the three low-energy polymorphs with an average deviation of less than 0.3 Å from experiment.
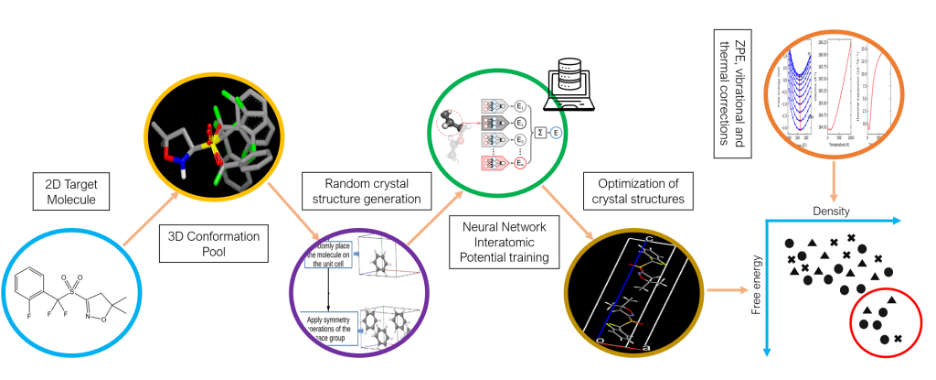
In the second phase the CMU team used system specific machine learned potentials to optimize the geometry and rank the relative stability of the structures provided by the CCDC. The relative stability of polymorphs can change depending on temperature. The team calculated thermal corrections to predict the relative stability at room temperature, which helped improve the ranking performance.
“I really enjoyed pushing the boundaries of our current crystal structure prediction workflow. What's great about the blind test is it really encourages, and almost requires, participants to develop robust and generalizable methods and really tests the limits of our current methods. It was rewarding to see that we did well in terms of our own expectations and that some of our newer methods worked,” Dana O’Connor explained.
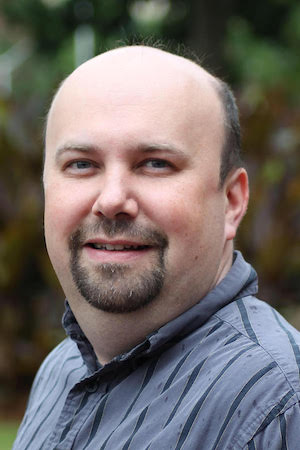
Olexandr Isayev
“In this challenge, we pushed the boundaries of computational chemistry too,” Isayev said. “Most of the current state-of-the-art methods use expensive quantum mechanical calculations. This limits applicability of CSP only to scientists with access to the largest supercomputers with millions of hours of resource allocations. Utilization of ML significantly democratizes the CSP process.”
“Moving forward, we need to re-examine all aspects of CSP and question which steps can be generalized or made autonomous,” Anstine claimed. “One of the issues with established approaches to CSP is there is an aspect of relying on the intuition of the chemist. While human accumulated insights are important, our ongoing efforts are looking at removing such dependencies, so we can start identifying crystal structures that are unfamiliar, complex, or perhaps unthinkable based on existing CSP experience.”
Marom said, “I am pleased with our team’s performance in the CSP blind test. It catapulted our method development and code implementation forward. This has been a major improvement compared to our performance in the previous blind test (with a very early version of our software). However, we still have a long way to go towards predicting the structure of more complex crystals, including hetero-molecular crystals (co-crystals, salts, and solvates) and crystals of flexible molecules. We look forward to participating in the next blind test.”